We’ve all heard the trope that you can make data say anything you want to. To a degree - it’s true. Researchers can (either intentionally or unintentionally) construct an environment where the sample or the reporting output is faulty and thus the results of the data are misleading or inaccurate.
The other side of the coin is that you can artfully design studies with the highest quality controls and data cleaning in such a way that the insights and recommendations can be trusted to guide the right business decisions.
Below are five examples from real market research studies commissioned from our custom market research firm by building products manufacturers and retailers.
What is the Data Cleansing Process?
Data cleansing is the process of both proactively preventing false respondents from entering a survey and retroactively scrubbing false respondents that may have found a way to take the survey. This 2-step process occurs before and after the survey is fielded.
During Survey Design & Fielding
Fielding the survey only to genuinely qualified respondents is the absolute bare minimum required to produce results with the highest accuracy. Your emphasis should be on preserving the quality of your study sample, not fixating on getting the largest sample size purely for the sake of having more. You must ensure your source of respondents is of the highest quality.
Your priority is to get accurate recommendations from the data, ensuring as best you can that the attributes of the population you are studying generally represents the broader population of homeowner or Pro customers who you are seeking to understand.
To do this, work with the research team to effectively design the survey using the proper respondent screening and data collection practices, including to design the right termination points into the survey, that will disqualify irrelevant participants from being included in the final results of the study. Our team uses over a dozen questions for screening and quality control in the design of our survey instruments.
Our team also invests in technology during survey fielding, designed to flag and toss out respondents that are not real based on a variety of factors such as IP address and digital profiling.
After Survey Fielding
While implementing proactive practices during survey design and fielding, the increasing sophistication of false responses means there is still data cleansing work to be done once survey fielding is closed to remove bad responses that made it through the survey.
Because of the incentives offered for completing market research or even the aim of disrupting your research efforts, marauders are keen to design programs that will impersonate a respondent to try and collect on these survey completion incentives. With the increasing availability of AI and spam programs, and the increasing pace at which these programs are becoming harder to distinguish from genuine human respondents, there’s a need for a second line of defense.
Going past the survey design and termination points and red herring questions that were designed into your survey as the first line of defense, the second line of defense is trained professionals that know your industry, your category, and your customer. Having human eyes look into each individual response, especially on open ended questions, is a time consuming but necessary step to ensure you have the highest data quality and therefore the most accurate insights and recommendations.
What are the methods of data cleaning?
Techniques used to cleanse data after survey fielding include the following:
Basic Data Cleansing Techniques
The basics of data cleansing are things all researchers can and should do:
- Cross checking the IP addresses to find duplicates.
- Checking for “speeders,” which are respondents that completed the survey too quickly.
- Checking for “straight-liners”, which are respondents that select the same value to each attribute in a grid question, producing a straight line of answers.
Advanced Data Cleansing Techniques
Advanced techniques are deployed by trained market research experts with deep industry, category, and customer experience to detect irregularities and suspect respondents. These techniques involve:
- Reviewing the open-ended responses to determine if they align with the question, known industry factors, the product category in question, and the respondent profile.
- Reviewing close-ended questions with limited correct answers based on industry fundamentals, category factors, and customer behaviors. Our team at The Farnsworth Group has over 300 years of combined industry expertise. We know how a variety of DIYers and Pros should respond, and when to question the legitimacy of survey responses.
- Reviewing similar questions with the survey to ensure responses are the same.
- Running calculations on numeric values provided by respondents to determine if they match prior responses and fall into ranges known to be true.
- Testing that the logic of a respondent is consistent throughout the survey and in line with known industry factors.
Uncleaned Data = Bad Corporate Strategies
Below is empirical evidence of the degree to which data quality impacts the actions your team may or may not take after getting the results from your research. Our team of market research experts at The Farnsworth Group have conducted thorough analysis across many projects.
We’ve selected five client projects to illustrate where the recommendations given to the client would have been wrong had our team not gone through our industry leading approach to data quality and cleansing, which is necessary to rid erroneous responses from the client’s data set.
Here’s what we found:
Note: Charts depicting “Basic Data Cleansing” show data that went through only the initial round of data cleansing that is considered the minimum practice by all researchers to reduce sample fraud using primarily automated and global tools and settings. Charts depicting “Advanced Data Cleansing” show data that went through The Farnsworth Group’s rigorous data quality and cleansing protocols: the standard initial round of data cleansing AND the second, manual round of data cleansing by our trained research experts to provide genuinely trustworthy results.
Example #1: How Bad Data Would Have Wasted Money on Media Investments & Missed the Ability to Reach a Qualified Audience
This client sought to understand how their leads were being acquired, with the intent to allocate marketing dollars based on the degree of influence various mediums were having on driving new leads.
“Referrals from past projects” is actually the most popular method of acquiring leads, not third, as indicated in the unclean data that only went through basic data cleansing. The unclean data indicates that “Social media advertising” is more important than it actually is for this client.
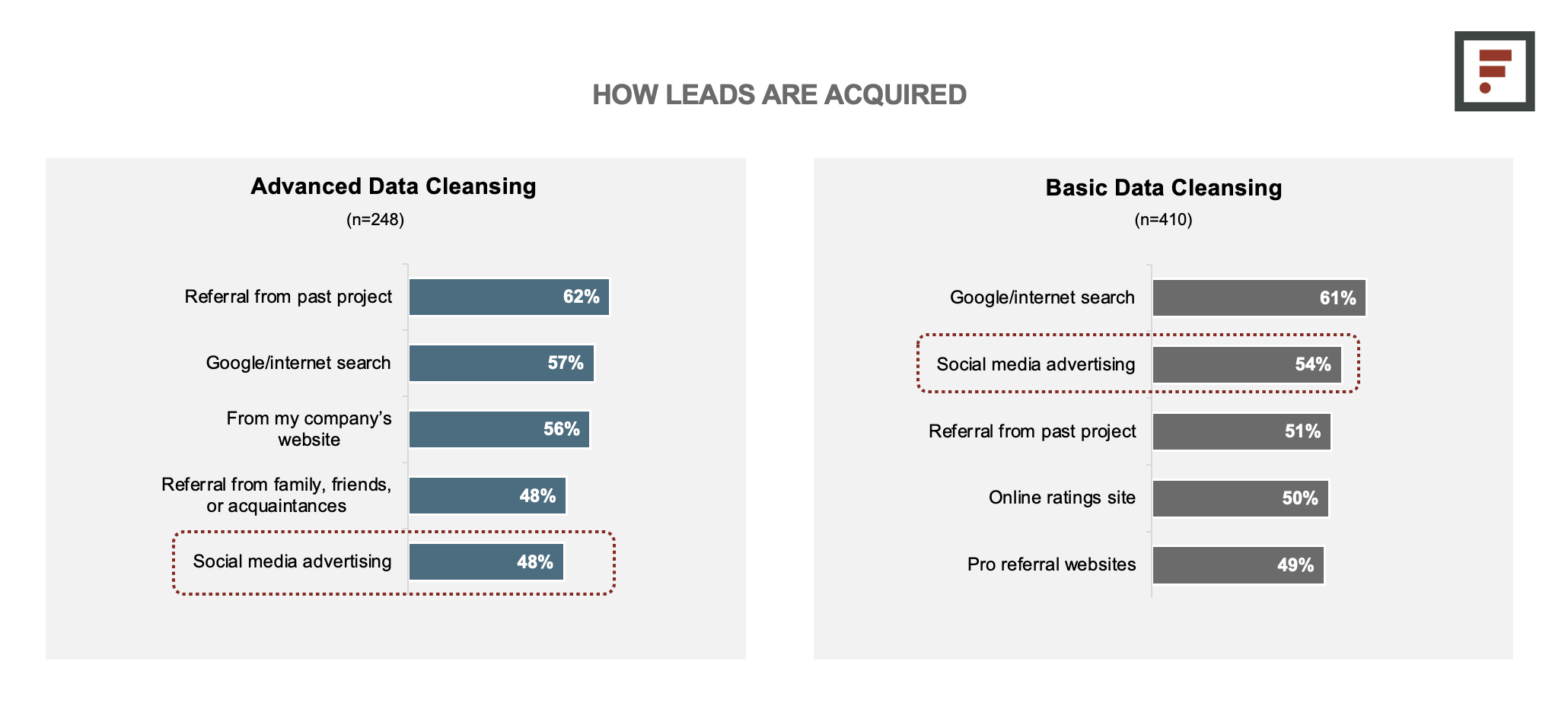
If only the first line of defense of data cleansing had been enacted, the recommendations would have been flawed, as social media advertising would have been falsely viewed as the second best source of leads. In reality, once the second phase of data cleansing was conducted, tossing out 162 of the 410 survey completes, the clean data revealed that social media advertising was not even in the top three sources of leads, and further, that referrals from past projects was actually the top source of leads.
If the bad data from the customer usage and attitude study had been presented to the client, their marketing budgets and efforts would have been falsely informed and invested into the wrong sources of leads, to the detriment of the company’s market share and unit sales.
Example #2: How Bad Data Supported Channel Distribution Strategies That Focused on the Wrong Suppliers, Costing Millions in Lost Sales
In this case, the client sought to understand the ways in which various building materials were being purchased by Pros customers.
Despite the basic requirements for data cleanliness standards implemented during survey design and fielding, nearly 50% of the responses that made it through the first line of defense were scrubbed before analysis and reporting were completed in order to adhere to The Farnsworth Group’s elevated standards of respondent qualification. This is because we know from decades of conducting market research studies that bogus respondents tend to select more responses per question, which skews the results, like in this instance.
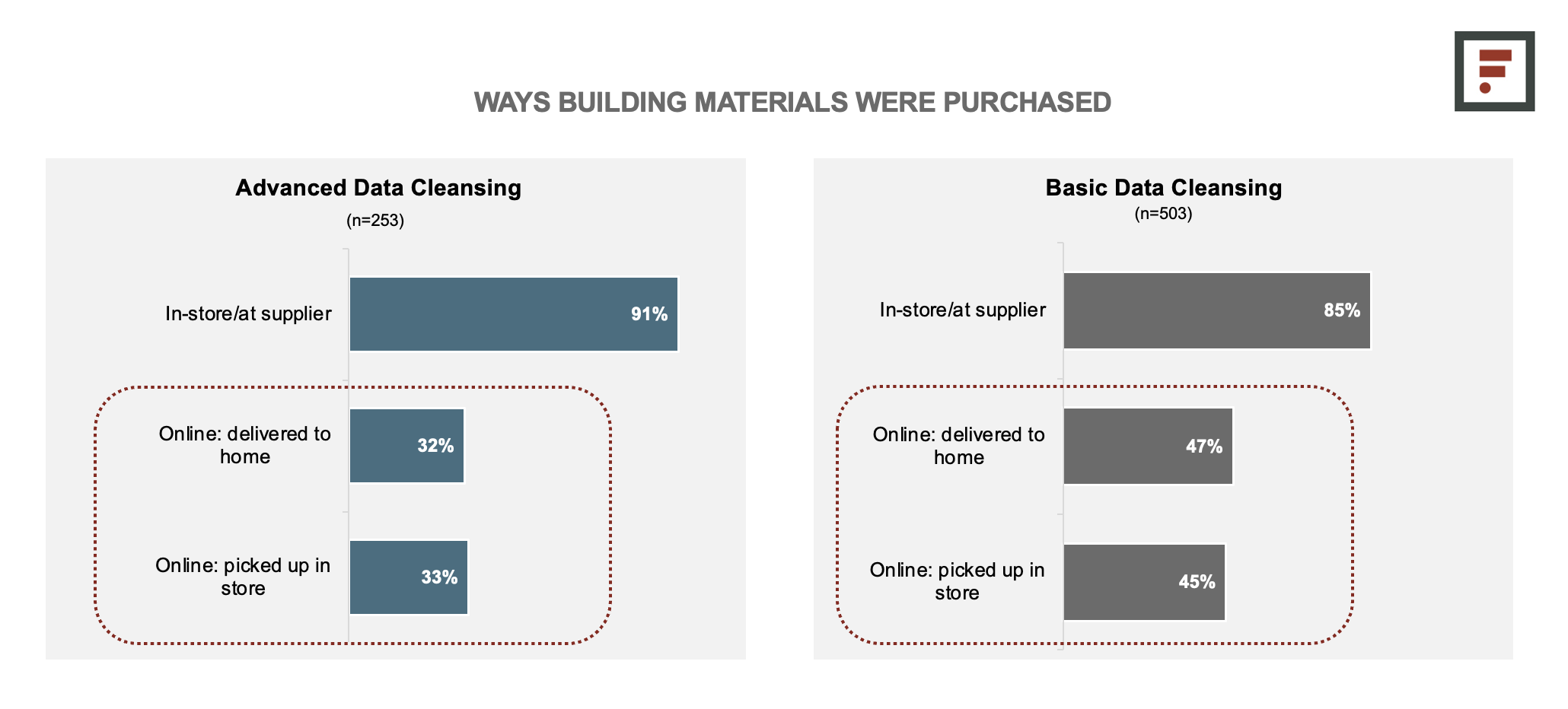
After the false responses were scrubbed, the study results indicated that only 1 in 3 shoppers were making online purchases, as opposed to the inflated suggestion from the bad data that 1 in 2 shoppers were making online purchases.
In other words, the data that was not thoroughly cleansed makes it appear that shoppers are purchasing those specific products online almost 50% more than they actually are.
Consider what your company would change about your channel strategy and go-to-market efforts if you thought that 50% more online purchases were being made than actually were! Under these false pretenses, that still seem believable due to eCommerce’s rising prevalence in the building materials industry, strategic distribution partnerships, built over decades, could have suffered far-reaching consequences overnight.
Limited corporate resources would have been improperly invested into eCommerce initiatives rather than channel partners and in-store displays and product placement efforts, costing millions in lost sales opportunities.
Example #3: How Marketing Budgets Would Have Been Improperly Allocated, and Measures Would be Wrong Based on Bad Data
Through our customized Brand Health research with our clients, aided brand awareness questions are used to identify which of the brands in the provided list customers associate with the question at hand.
In the brand health study example below, you can see how the efforts taken to preserve the accuracy of the data set reduced the sample size analyzed from 1,699 to just 605 genuine respondents.
Suffice it to say that more is not always better.
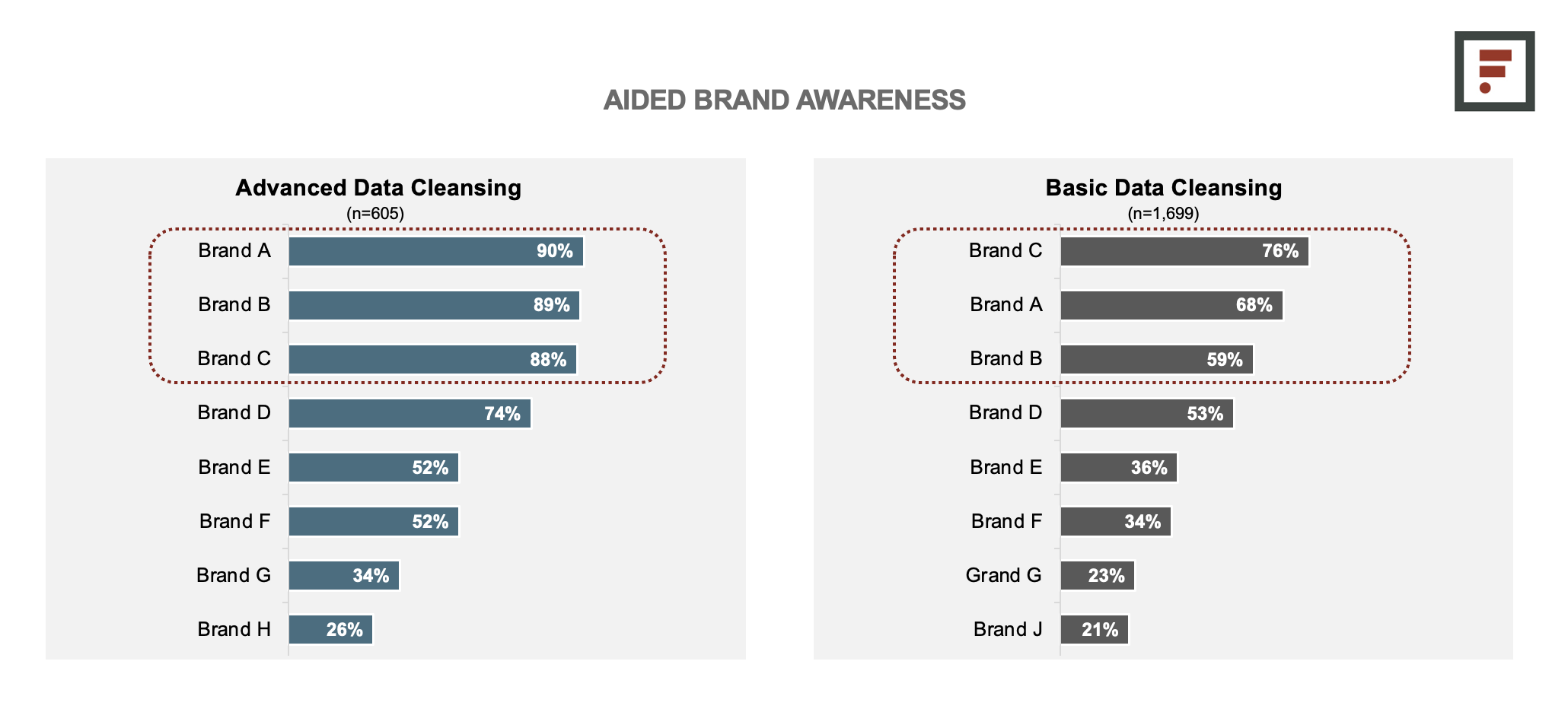
For this client, had our analysts at The Farnsworth Group not gone through the process to remove false data, the recommendations provided would have suggested a significantly lower degree of brand awareness for each brand, as well as an entirely different ranking order.
Had this improper data been presented to the client, they would have proceeded to invest more dollars into brand awareness campaigns than were actually necessary, thereby wasting limited marketing dollars that would have been better spent elsewhere.
Beyond wasting money, the marketing team would be sharing wrong metrics with their channel partners, impacting their supplier’s decision about which brands to carry and which to remove. A major implication that would affect sales across the entire category. Not to mention, the manufacturer losing credibility with their supplier.
We also see this in understanding how the percentage of brands installed in the past 12 months is widely different after the second round of data cleansing performed by The Farnsworth Group.
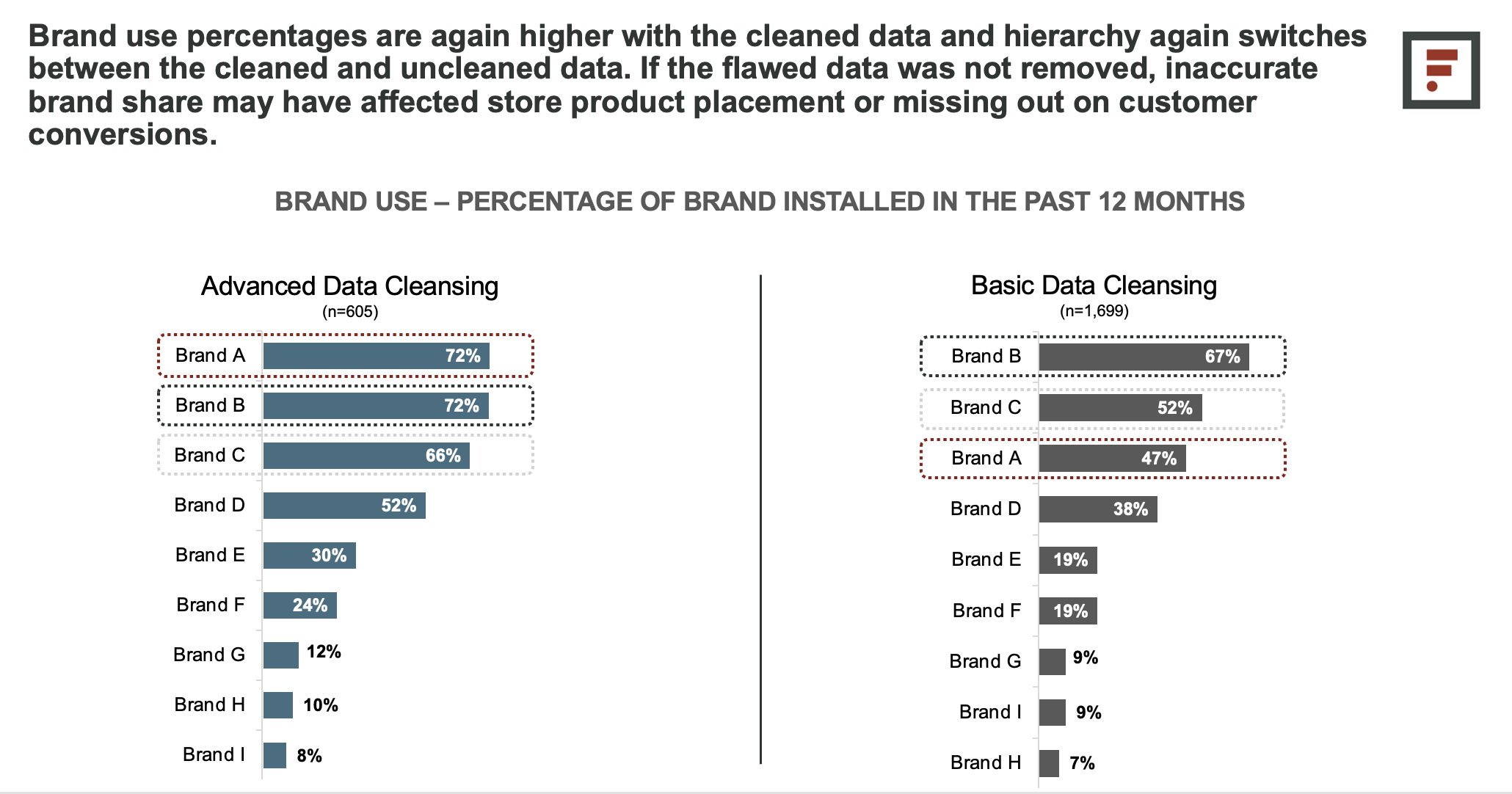
Brand use percentages are again higher with the cleaned data and hierarchy again switches between the cleaned and uncleaned data. If the flawed data was not removed, inaccurate brand share may have affected store product placement or missing out on customer conversions.
Further, the spread between top brands used is smaller than the flawed data suggests, signally a much more competitive environment for these brands to operate within.
Example #4: How Bad Data About Product Usage Would Have Mislead Teams to Make Wrong Decisions on What to Make, Where to Sell, How to Market
Here’s another example of how unclean data would have resulted in misguided recommendations for how to allocate business resources. In this case, the sample size of professional contractors was reduced from 450 to 191 by removing fraud in the online sample in order to preserve the accuracy of the analysis and recommendations.
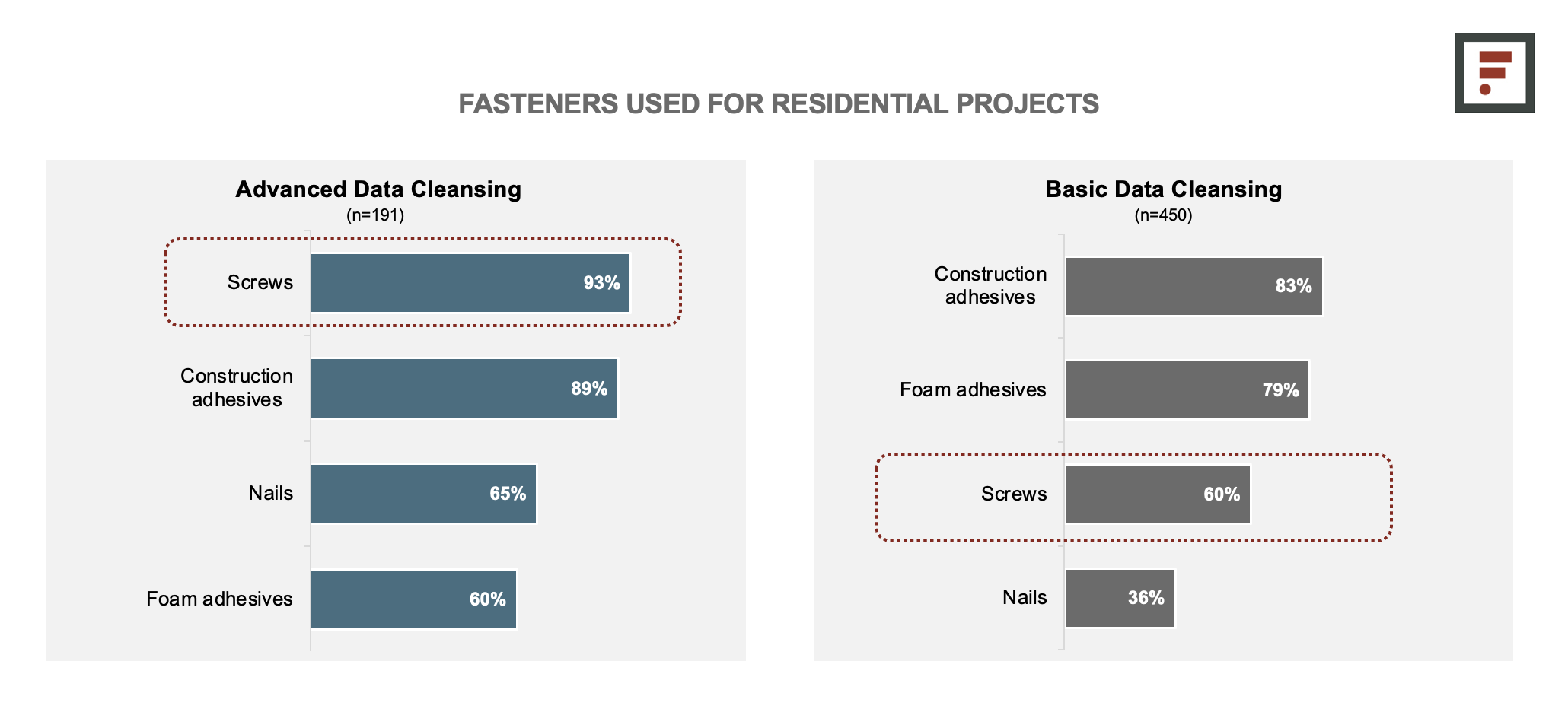
The bad data suggests that Pros are using all versions of the fasteners less than they actually are. Further, the bad data would have suggested that Pros prefer using construction adhesives and foam adhesives to using screws and nails. The clean data shows that that premise is entirely unfounded, and to a great degree!
The differences in study results don’t stop there. Consider the following charts:
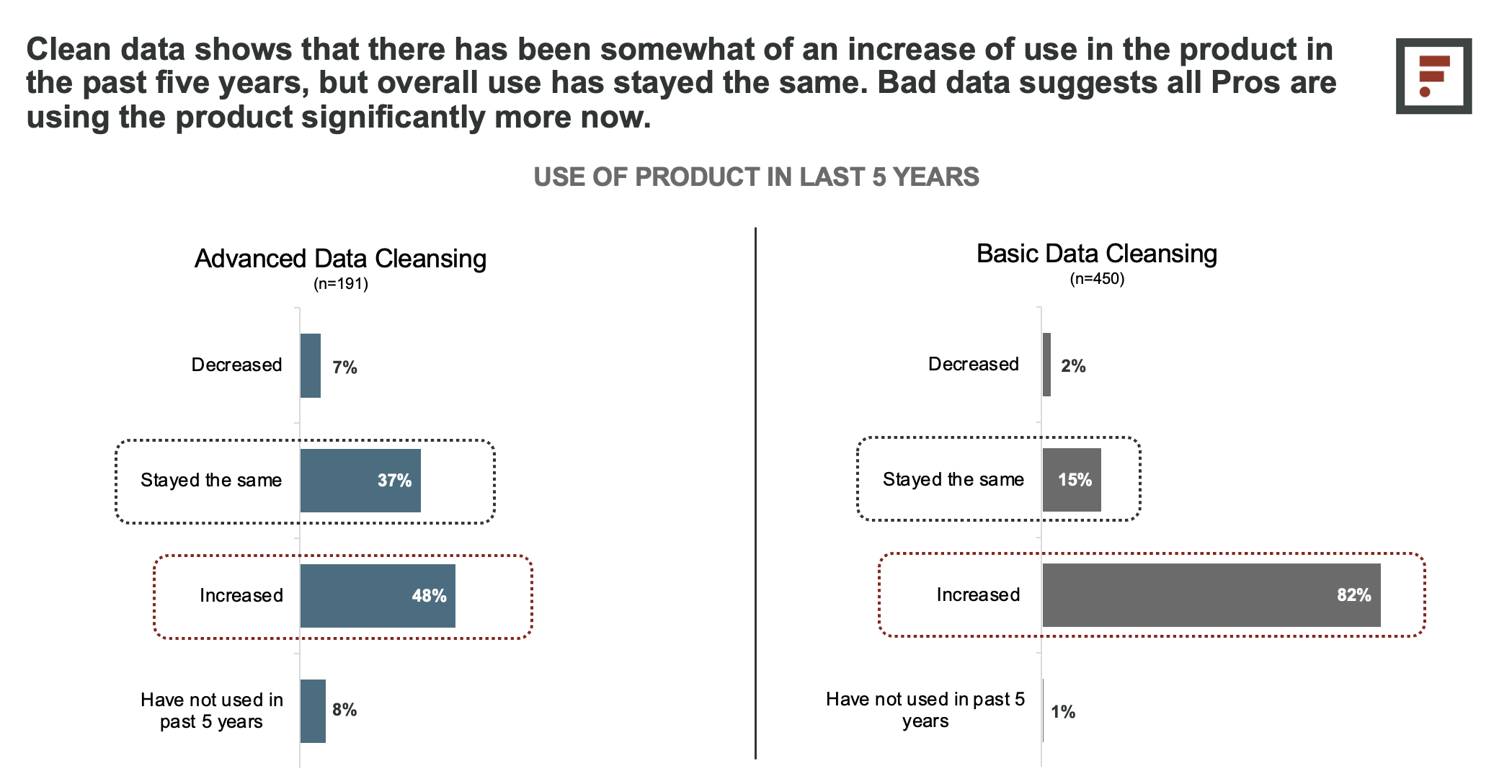
Clean data provided by The Farnsworth Group shows that there has been a degree of increased use in this product in the past five years, but that for many customers, overall use has stayed the same. This is in contrast to bad data that suggests 82% of Pros are using the product more in the last 5 years, when the clean data shows just 48% of Pros are using the product more. Further, 7% of Pros are using the product less and 8% of Pros have not used the product at all in the last 5 years based on analysis of the fully cleaned data. The flawed data set suggested a different story, of nearly universal use among Pros and barely any decrease in product usage.
This flawed data would guide product, channel, and marketing teams to make completely wrong decisions that would negatively impact their business for years to come. Bad data would cause an over investment into the wrong product with high sales expectations. Product development takes millions of dollars and many months of staff resources, and it all would have been invested incorrectly. Thankfully, our team’s expertise was able to provide our client with reliable data to make the best strategic decisions needed for future growth.
Example #5: How Bad Data Can Affect Demand Forecasting and Market Share Benchmarking
When it comes to conducting brand health research, getting accurate data that can be repeatedly benchmarked against proves to be challenging for many manufacturers. This is because sample quality is the biggest driver of results.
Especially when fielding a study among homeowners with a high incidence rate, the second, intense round of data cleansing is critical to ensure each respondent is genuinely representative of the target population.
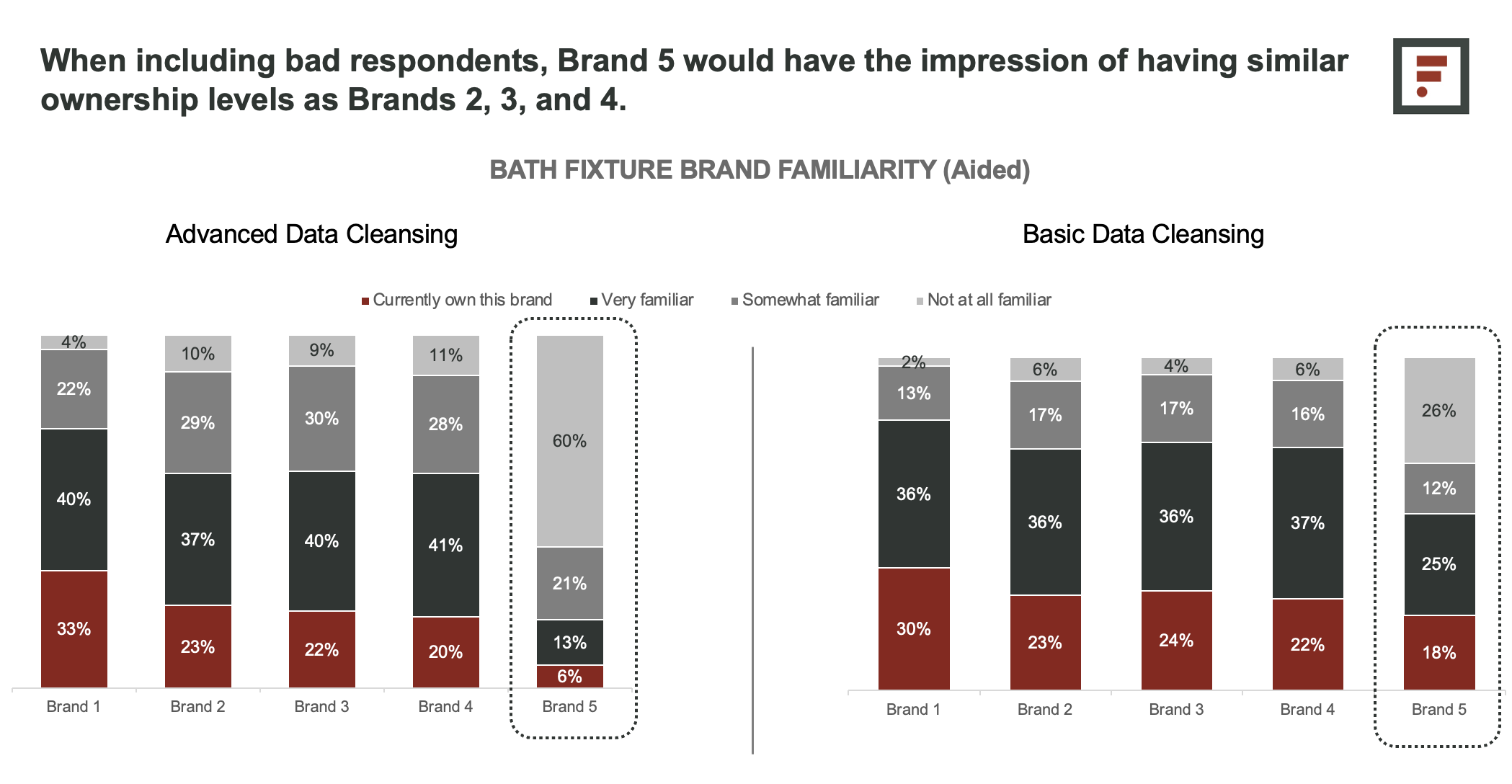
The Farnsworth Group put in the effort to reduce the data sample analyzed by 6x because our researchers and analysts operate under the premise of, “If in doubt, toss it out,” to preserve the accuracy of the data and the veracity of the recommendations we are providing to clients.
It's apparent how Brand 5 would have the impression of having similar ownership levels as Brands 2, 3, and 4 without implementation of advanced data cleaning. Further, Brand 5’s ownership would have been overstated by 300%.
If the data on the right had been presented to the client, they would have been falsely informed about their true standing against competitor brands in the category. These skewed perceptions of market share would have had negative consequences on corporate strategy and demand forecasting. The clean data, and thus knowing the true brand share of competitors of bidet seats among homeowners, illuminated market opportunities for growth for this client.
Don’t Stake Your Decisions on Bad Data
When you schedule a consultation with our building products research team, you’ll be talking with a team that genuinely cares to get you the most accurate findings from the audience you need to get answers from.
We’re not interested in providing you with a false sense of security in larger sample sizes; you will always be able to find an alternative research firm or data source that touts their ability to get you a larger sample size than what our team will commit to. That’s because their standards for data accuracy are lower than ours here at The Farnsworth Group.
When working to understand who your customers are, why they are making the choices they make, and how to influence their purchase decisions, we stand by providing building products manufacturers and retailers with accurate insights above all else.
Curious if you can trust the data your teams are basing their decisions off of? Schedule a consultation with our industry experts to get a sense of how the markets are shaping up from our vantage point.
The Farnsworth Group has developed customized research methods designed specifically to serve the Building, Home Improvement, and Lawn & Ranch industries. Driving successful strategies often means gaining deeper insights on four critical areas: Customer, Brand, Product and Market. We have decades of industry specific research expertise to craft research that addresses your specific needs - resulting in actionable recommendations for you to make accurate and informed strategic decisions.